In the age of Industry 4.0, industrial firms are increasingly dependent operational data like time series data to oversee and enhance your operations. Yet, the management and analysis of this data present distinct challenges. This discussion delves into the five most crucial data quality challenges that industrial companies encounter with time series data, drawing on insights from multiple sources.
It’s often said that data is as precious as gold. That sounds promising, but what if your gold is so fine it slips through your fingers? The value of data lies in its governance, completeness, and reliability. Let’s dive into the different aspect you could think about.
Tackling incomplete time series data in the industrial segment.
Filling data gaps for accurate trend analysis.
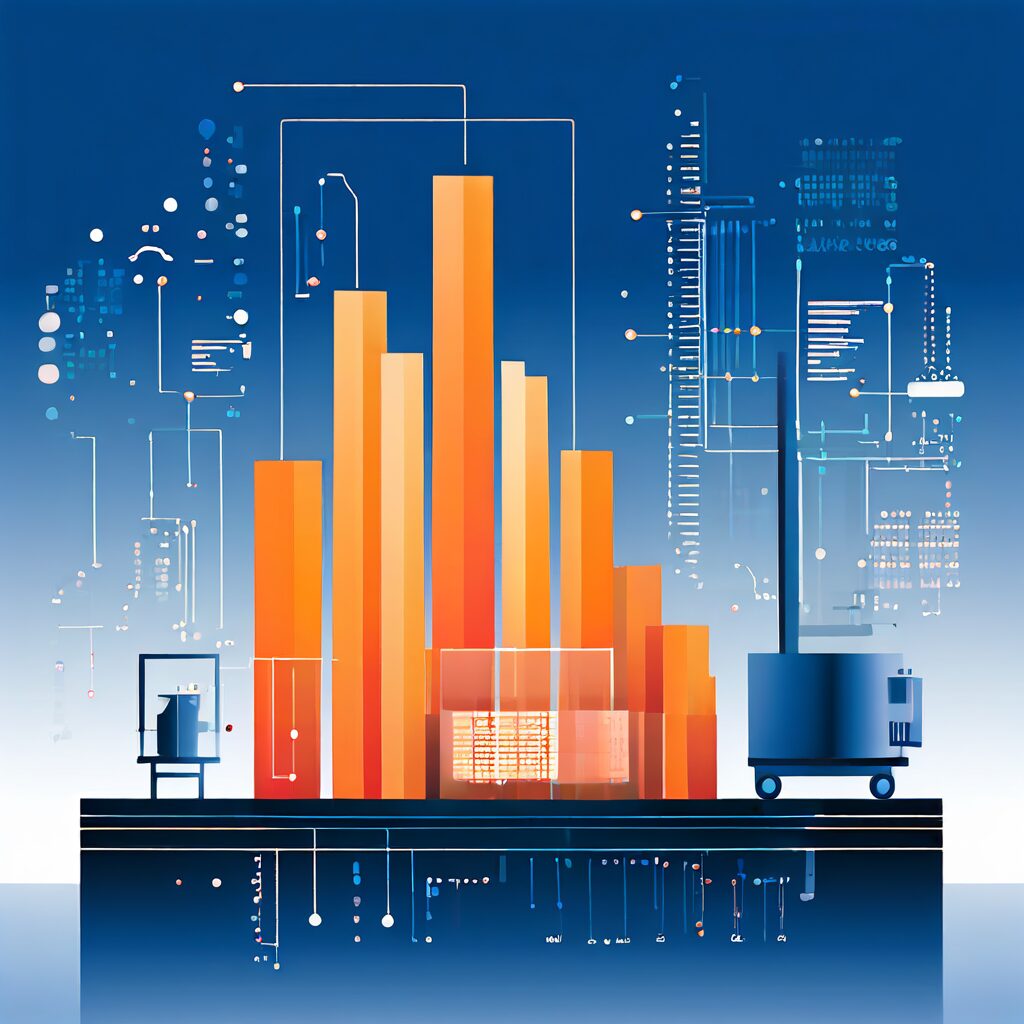
Incomplete data can significantly hinder the ability to analyze trends and patterns accurately. For instance, missing values in sensor data can lead to incorrect predictions and operational inefficiencies. A manufacturing company faced challenges due to gaps in their production data, which affected their ability to optimize processes. By implementing automated data collection systems and integrating IoT sensors, they were able to capture comprehensive data, leading to a 15% increase in production efficiency.
Solution:
Techniques such as data imputation and machine learning algorithms can be employed to predict and fill missing values. Tools like Timeseer.AI can help detect and address data quality issues before they impact operations.
Leveraging data enrichment techniques.
Data enrichment involves supplementing incomplete data with additional information from external sources. A logistics firm used third-party data to fill gaps in their shipment tracking information, improving their delivery accuracy by 20%. This approach ensures that the data is as complete and accurate as possible, enabling better decision-making.
Solution:
Data enrichment tools and platforms like Azure Time Series Insights can integrate various data sources to provide a more complete dataset.
Standardizing time series data in industrial systems.
Standardizing data formats for seamless integration.
Inconsistent data formats can cause integration issues and data misinterpretation. A chemical manufacturing company standardized their data formats across all systems, which reduced data processing time by 30%. Implementing data standards and using middleware solutions can help achieve consistency.
Solution:
Establishing data governance policies and using tools like Collibra and Informatica Data Quality can ensure consistent data management practices.
Implementing data governance frameworks.
Data governance frameworks ensure consistent data management practices. A global automotive supplier adopted a data governance framework that included data quality rules and regular audits, resulting in a 25% improvement in data accuracy and consistency.
Solution:
Data governance tools and frameworks can help maintain data consistency across various systems and processes.
Combating data inaccuracy in industrial analytics.
Implementing Real-Time Data Validation.
Real-time data validation helps in identifying and correcting inaccuracies as data is collected. A food processing company implemented real-time validation checks on their sensor data, reducing errors by 40%. Techniques include rule-based validation and anomaly detection using machine learning.
Solution:
Tools like Alteryx for data validation and UiPath for robotic process automation (RPA) can help maintain data accuracy.
Enhancing Data Accuracy with Automated Systems.
Automation reduces human errors and improves data accuracy. A pharmaceutical company automated their data entry processes, which led to a 35% reduction in data inaccuracies. Automated systems can include robotic process automation (RPA) and AI-driven data entry tools.
Implementing automated data entry systems and real-time validation protocols can significantly enhance data accuracy.
Ensuring data uniqueness in industrial operations.
Implementing Master Data Management (MDM) for industrial companies and time-series data
Master Data Management (MDM) is a critical process for ensuring the accuracy, consistency, and completeness of data across an organization. While MDM is often associated with IT systems, its principles and benefits are equally applicable to Operational Technology (OT) systems in industrial settings. Industrial companies generate vast amounts of time-series data from sensors, machines, and other IoT devices. Managing this data effectively requires robust MDM practices to ensure data quality and facilitate meaningful analysis.
Opportunities:
Unified data view: Implementing MDM can help create a single source of truth for time-series data, integrating it with other business data to provide a holistic view of operations.
Improved decision-making: High-quality, consistent data enables better predictive maintenance, operational efficiency, and strategic planning.
Regulatory compliance: MDM ensures that data is accurate and up-to-date, helping companies comply with industry regulations and standards.
Examples of concepts
A manufacturing company faced challenges with fragmented data from various sensors and machines. By implementing an MDM solution, they were able to consolidate this data into a single, authoritative source. This integration allowed them to perform advanced analytics, leading to a 20% reduction in machine downtime through predictive maintenance.
An energy company used MDM to integrate time-series data from their power plants with their ERP system. This integration provided real-time insights into energy production and consumption, enabling more efficient energy management and reducing operational costs by 15%.
Solution:
Tools like Informatica MDM and Talend Data Quality can help manage and maintain unique data records.
Xenonstack: Uses MDM in manufacturing for managing master data and improving data quality, which can lead to better decision-making and operational efficiency
Sphera : MDM can improve decision-making and operational performance by managing data from maintenance, procurement, and supply chain systems.
Using Data Deduplication Techniques.
Data deduplication helps in identifying and removing duplicate records. A telecommunications company used deduplication algorithms to clean their customer database, improving data quality by 30%. Techniques include fuzzy matching and clustering algorithms.
Solution: Implementing deduplication routines and using tools like Talend Data Quality can help maintain data uniqueness.
Managing operational data obsolescence in industrial systems.
Implementing operational data lifecycle management
Operational data lifecycle management ensures that data is regularly reviewed and obsolete data is archived or deleted. A financial services company implemented a data lifecycle management policy, reducing storage costs by 20%. This involves setting retention policies and automated archiving processes.
Solution: Tools like Varonis for data lifecycle management and audit can help manage data obsolescence.
Regular operational data audits to identify obsolete data
Regular data audits help in identifying and managing obsolete data. A healthcare provider conducted quarterly data audits, which improved data relevance and reduced clutter by 25%. Audits involve reviewing data usage and relevance periodically.
Solution: Establishing regular audit schedules and using audit checklists can help maintain data relevance and reduce obsolescence.
Conclusion: The critical importance of reliable data in the industrial sectors.
In the industry, the next step of maturity is the use of smart agents powered by Artificial Intelligence (AI) represents a paradigm shift towards more efficient, autonomous, and intelligent systems. These AI models promise to revolutionize the industry by optimizing production processes, reducing costs, and enhancing product quality.
However, the foundation of these transformative benefits rests upon the reliability and integrity of the data fed into these models. The significance of employing trustworthy data in the development and operation of AI models for smart agents in manufacturing cannot be overstated.
The consequences of unreliable operational data
Utilizing unreliable or questionable data in AI models for manufacturing can lead to several detrimental outcomes, which include:
- Erroneous decision-making: AI models are only as good as the data they analyze. If the data is flawed, the decisions made by these models will be equally flawed, leading to operational inefficiencies and potentially costly mistakes.
- Compromised model effectiveness: The accuracy and reliability of AI which, are predictions, are directly tied to the quality of the data. Poor data quality can severely hinder the model’s ability to make accurate predictions, rendering it less effective or even useless.
- Wasted resources: Implementing AI models in manufacturing requires significant investment in terms of time, money, and human resources. Utilizing unreliable data can render these investments fruitless, as the outcomes will not meet the expected standards.
- Loss of competitive edge: In the highly competitive manufacturing sector, the ability to leverage AI effectively can provide a significant advantage. Relying on questionable data can negate this advantage, leaving companies struggling to keep up with more data-savvy competitors.
The importance of trustworthy operational data
To avoid these pitfalls and ensure the successful implementation and operation of AI models in the manufacturing industry, it is imperative to prioritize the acquisition, verification, and maintenance of high-quality, trustworthy operational data. This involves:
- Implementing robust data management practices: Establishing strong data governance, quality control measures, and regular audits to ensure the integrity of the data.
- Sourcing data responsibly: Obtaining data from reliable sources and ensuring it is relevant, accurate, and up-to-date.
- Continuous monitoring and improvement: Regularly evaluating the data’s impact on model performance and making necessary adjustments to maintain data quality over time.
In conclusion, the promise of AI in revolutionizing manufacturing through smart agents is undeniably compelling. However, the realization of this promise hinges on the quality of the data that these models rely on. Unreliable data can significantly hinder the effectiveness of AI models, leading to poor decision-making, wasted resources, and lost opportunities.
Conversely, investing in trustworthy data ensures that the AI models function as intended, delivering the expected outcomes and securing a competitive advantage in the dynamic manufacturing landscape. Invest smartly is the message and don’t miss a step in your process
FAQ
What are the challenges in managing time operational data in the industrial sector?
The management of time series data in the industrial sector presents challenges such as incomplete data, inconsistent data formats, data inaccuracy, data duplication, and data obsolescence. These issues can hinder trend analysis, cause integration issues, compromise data accuracy, and lead to inefficient data management practices.
What are the benefits of data enrichment techniques of operational data in the industrial sector?
Data enrichment techniques supplement incomplete data with additional information from external sources, ensuring that the data is as complete and accurate as possible. This enables better decision-making and can improve operational efficiency. Tools like Azure Time Series Insights can integrate various data sources to provide a more complete dataset.
What is the role of data governance frameworks in managing operational data or time series data?
Data governance frameworks ensure consistent data management practices. They include data quality rules and regular audits, which can lead to improvements in data accuracy and consistency. Data governance tools and frameworks can help maintain data consistency across various systems and processes.