In today’s data-driven world, defining clear objectives for managing operational data or time series data is paramount for any industrial company aiming to achieve excellence. The importance of setting these objectives cannot be overstated, as they form the foundation for effective data management, enabling organizations to optimize their operations, enhance decision-making, and maintain a competitive edge.
Operational data encompasses a wide range of information, from raw data collected through various data sources to historical data that provides insights into past performance. By establishing well-defined objectives, companies can streamline their data collection processes, ensuring that the data is accurate, relevant, and timely. This is critical for maintaining high data quality, which is essential for making informed decisions and driving business intelligence initiatives.
Data science and machine learning play a crucial role in analyzing data and uncovering valuable insights. By leveraging these technologies, data scientists and analysts can compute complex metrics and key performance indicators, helping organizations to optimize their supply chain, reduce costs, and improve customer satisfaction. The ability to analyze unstructured data and visualize it effectively through data visualization tools further enhances the decision-making process.
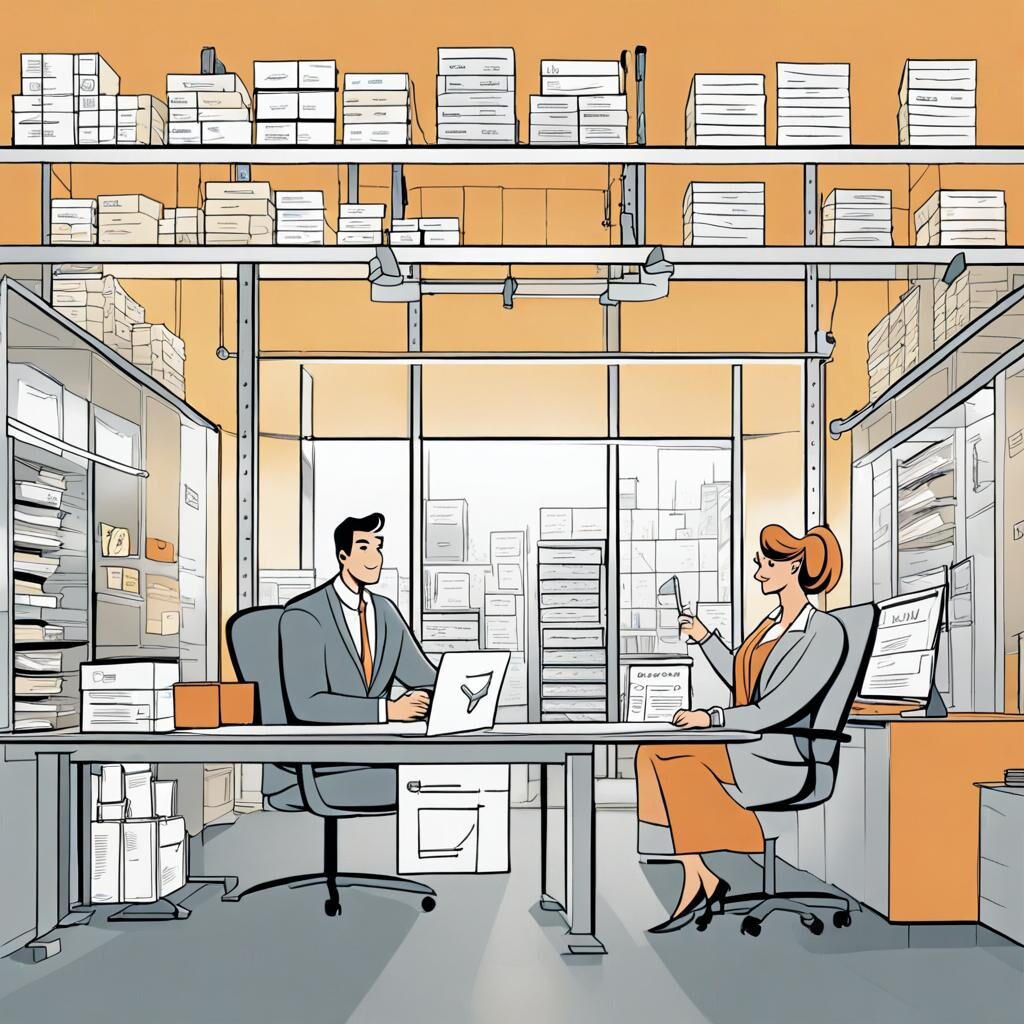
Implementing best practices in data management ensures that the management system is robust and capable of handling the vast amount of data generated by modern industrial operations. This includes ensuring data privacy and security, which are non-negotiable aspects of any data management strategy. By adhering to these best practices, companies can build a reliable and efficient data management system that supports their business processes and meets the needs of all stakeholders.
To summarize: defining clear objectives for managing operational data or time series data is not just a best practice; it is a critical, non-negotiable step for any industrial company aspiring to lead in operational data management. By setting these objectives, organizations can streamline their data processes, make informed decisions, and ultimately achieve excellence in their operations.
Setting clear objectives
Setting clear objectives is a fundamental step in managing operational data or time series data effectively. These objectives align data management efforts with business goals, ensuring that every data point collected and analyzed contributes to the organization’s strategic planning and operational efficiency. Clear objectives provide a roadmap for data analysts and data scientists, guiding their efforts in data analysis, trend analysis, and the application of time series analysis techniques. This alignment helps in making informed decisions based on historical data and trend analysis, ultimately optimizing business processes and enhancing overall performance.
Using the SMART framework to set objectives ensures they are Specific, Measurable, Achievable, Relevant, and Time-bound. This approach provides clarity and focus, making it easier to track progress and measure success. For example, a specific objective might be to “reduce data entry errors by 20% within six months through enhanced training and validation processes.” This objective is measurable, achievable with the right resources, relevant to improving data quality, and time-bound with a clear deadline. By setting such SMART objectives, companies can ensure that their data management practices are aligned with broader business goals and are actionable within a given timeframe.
The people aspect of the organization is also crucial in setting and achieving clear objectives. Engaging stakeholders, including data analysts and data scientists, in the objective-setting process ensures that their insights and expertise are leveraged effectively. Training and development programs can enhance the skills of team members, enabling them to handle big data and perform effective data analysis. Collaboration and communication are key, fostering a culture where team members can share insights and best practices. Clear objectives are non-negotiable for becoming a leader in operational data management, providing a competitive advantage through superior data governance, data security, and data analysis.
Best practices in data management
Effective data management is crucial for any organization aiming to leverage its data assets for strategic advantage. Establishing clear and achievable objectives is a fundamental step in this process, ensuring that data management efforts align with business goals and drive operational efficiency. Here are some best practices to guide you in setting and achieving these objectives.
Establishing a data governance framework
A robust data governance framework is essential for managing data from various sources and ensuring its quality and integrity. This framework should define roles, responsibilities, and processes for handling business data, including data from various departments and systems. By setting clear guidelines and standards, organizations can streamline their data management process, making it easier to collect, store, and analyze relevant data. This approach not only enhances the availability of data but also ensures that it is used effectively across the organization.
Ensuring data quality from the onset
Data quality is a critical component of any data management strategy. Ensuring high data quality from the onset involves implementing data cleansing and data validation processes to remove inaccuracies and inconsistencies. This is particularly important for operational data and time-series analysis, where the accuracy of data points can significantly impact the outcomes of the analysis process. Regular data cleaning and validation help maintain the reliability of the data platform, enabling better decision-making and more accurate insights.
Implementing data lifecycle management
Data lifecycle management (DLM) is a comprehensive approach to managing data throughout its lifecycle, from creation to deletion. This involves setting policies and procedures for data collection, storage, usage, archiving, and disposal. By implementing DLM, organizations can ensure that data is managed efficiently and remains relevant throughout its lifecycle. This includes managing different data types and ensuring that new data is integrated seamlessly into the existing data architecture. Effective DLM also supports inventory management and database management, ensuring that data is always available and accessible when needed.
Prioritizing data security and privacy
Data security and privacy are paramount in today’s data-driven world. Organizations must prioritize the protection of their data assets by implementing robust security measures and privacy policies. This includes securing data from various sources, ensuring compliance with regulatory requirements, and protecting sensitive information from unauthorized access. By prioritizing data security and privacy, organizations can build trust with stakeholders and ensure the safe handling of business data.In conclusion, setting clear and achievable objectives in data management is critical for becoming a leader in operational data management. By establishing a data governance framework, ensuring data quality, implementing data lifecycle management, and prioritizing data security and privacy, organizations can effectively manage their data assets and drive strategic success
Analysis techniques for time series data
Effective analysis techniques for time series data are essential for any organization aiming to leverage its data for strategic advantage. Setting clear and achievable objectives is crucial in this context, ensuring that data management efforts align with business goals and drive operational efficiency. Here are some key analysis techniques and their importance in managing time series data.
Overview of time series analysis techniques
Time series analysis involves various techniques to uncover patterns and make predictions based on historical data. Some of the most widely used techniques include:
- ARIMA (Auto Regressive Integrated Moving Average): This model is used for forecasting and understanding time series data by capturing different aspects like trend and seasonality.
- Exponential Smoothing: This technique is useful for making short-term forecasts by smoothing out fluctuations in the data to highlight trends and patterns.
- Decomposition Models: These models break down a time series into trend, seasonal, and irregular components, making it easier to analyze and interpret the data.
These techniques are fundamental in data science and data management, providing a robust framework for analyzing time series data and making data-driven decisions.
Importance of trend analysis and seasonality detection
Trend analysis and seasonality detection are critical components of time series analysis. Identifying trends helps organizations understand the long-term direction of their data, whether it is increasing, decreasing, or remaining constant. Seasonality detection, on the other hand, uncovers recurring patterns that occur at regular intervals, such as monthly sales spikes or seasonal demand fluctuations.
- Trend Analysis: Helps in strategic planning by providing insights into long-term patterns and enabling better decision-making.
- Seasonality Detection: Allows organizations to optimize their operations by anticipating periodic fluctuations, improving inventory management, and enhancing customer satisfaction.
By incorporating these analysis techniques, organizations can streamline their management process and ensure that their data management system is aligned with their business objectives.
Using time series analysis for predictive maintenance and supply chain optimization
Time series analysis is particularly valuable for predictive maintenance and supply chain optimization. By analyzing historical data, organizations can predict equipment failures and schedule maintenance proactively, reducing downtime and improving operational efficiency. Similarly, in supply chain management, time series analysis helps in forecasting demand, optimizing inventory levels, and ensuring timely delivery of products.
- Predictive maintenance: Uses time series data to anticipate equipment failures and schedule maintenance, thereby minimizing downtime and reducing costs.
- Supply chain optimization: Enhances decision-making by predicting demand patterns, optimizing inventory, and improving logistics.

These applications of time series analysis are critical for maintaining a competitive edge in today’s data-driven business environment. By leveraging best practices in data analysis and employing advanced time series analysis techniques, organizations can achieve greater efficiency, improve customer satisfaction, and drive overall business success. In conclusion, setting clear objectives and employing robust analysis techniques for time series data are essential for becoming a leader in operational data management. These practices enable organizations to make informed decisions, optimize their operations, and maintain a competitive advantage in their respective industries.
Enhancing customer satisfaction through data management
Effective data management is crucial for enhancing customer satisfaction, as it allows organizations to leverage their data assets to improve product quality and customer service. By using data from various sources, including structured and unstructured data, companies can gain valuable insights into customer needs and preferences. This information from the data can be used to make informed decisions that enhance the overall customer experience.
Using data to improve product quality and customer service
Data management enables organizations to collect and analyze data across different touchpoints, ensuring that they have access to data that is accurate and relevant. For instance, sensor data and customer feedback can be analyzed to identify areas where products or services need improvement. By implementing time series analysis techniques, companies can track the sequence of data points over time to detect patterns and trends that inform product development and service enhancements. This type of analysis helps ensure that the data used is actionable and leads to tangible improvements in customer satisfaction.
Examples of how data analysis can lead to better customer satisfaction
Data analysis plays a pivotal role in identifying and addressing customer pain points. For example, by analyzing past data related to customer interactions, companies can pinpoint common issues and develop solutions to prevent them in the future. Database management systems can store and organize this data, making it easier for data analysts to perform detailed analysis. Additionally, feedback loops are essential in this process, as they allow organizations to continuously collect and act on customer feedback. This iterative approach ensures that improvements are based on actual data and customer experiences, leading to higher satisfaction levels.
Importance of feedback loops in operational data management
Feedback loops are a critical component of effective data management, as they provide a mechanism for collecting, analyzing, and acting on customer feedback. By integrating feedback loops into their data management strategies, organizations can ensure that they are responsive to customer needs and can make data-driven decisions that enhance customer satisfaction. This involves setting up systems to capture feedback, performing regular analysis to identify trends, and implementing changes based on the insights gained. The continuous cycle of feedback and improvement helps organizations maintain high standards of customer service and operational efficiencies.
Remember, enhancing customer satisfaction through data management involves using analytics and data science to improve product quality and customer service, leveraging feedback loops to drive continuous improvement, and ensuring that data is accurate and actionable. By focusing on these areas, organizations can become leaders in customer satisfaction, ultimately driving business success and customer loyalty.
Recap of the importance of clear objectives in data management
Clear objectives provide a roadmap for data analysts and data scientists, guiding their efforts in data analysis, trend analysis, and the application of time series analysis techniques. This alignment helps in making informed decisions based on historical data and trend analysis, ultimately optimizing business processes and enhancing overall performance. Setting clear objectives is non-negotiable for becoming a leader in operational data management, providing a competitive advantage through superior data governance, data security, and data analysis.
Summary of best practices and techniques discussed
We discussed several best practices in data management, including establishing a robust data governance framework, ensuring data quality from the onset, implementing data lifecycle management, and prioritizing data security and privacy. These practices help manage data from various sources, ensuring that it is accurate, relevant, and secure. Additionally, we explored analysis techniques for time series data, such as ARIMA, exponential smoothing, and trend analysis, which are critical for predictive maintenance and supply chain optimization. These techniques enable organizations to make data-driven decisions and optimize their operations.
Final thoughts on the future of operational data management in industrial companies
The future of data management in industrial companies will be shaped by advancements in data science, machine learning, and artificial intelligence. As data becomes an increasingly valuable asset, organizations must continue to refine their data management strategies to stay competitive. This includes adopting new technologies, enhancing data governance practices, and fostering a data-driven culture within the organization. By setting clear objectives and following best practices, industrial companies can harness the full potential of their data, driving innovation, improving customer satisfaction, and achieving long-term success.